M3Cam: Extreme Super Resolution via Multi-Modal Optical Flow for Mobile Cameras
Nov 2, 2024·,,,,,,,,,·
0 min read
Yu Lu
Hao Pan* (Corresponding Author)
Dian Ding
Yongjian Fu
Liyun Zhang
Feitong Tan
Ran Wang
Yi-Chao Chen
Guangtao Xue
Ju Ren
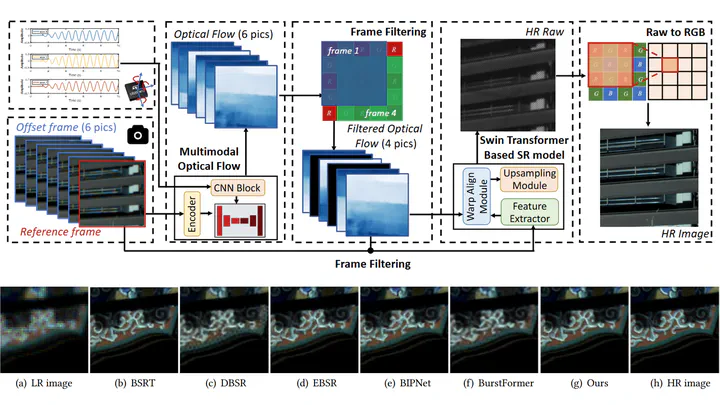
Abstract
The demand for ultra-high-resolution imaging in mobile phone photography is continuously increasing. However, the image resolution of mobile devices is typically constrained by the size of the CMOS sensor. Although deep learning-based super-resolution (SR) techniques have the potential to overcome this limitation, existing SR neural network models require large computational resources, making them unsuitable for real-time SR imaging on current mobile devices. Additionally, cloud-based SR systems pose privacy leakage risks. In this paper, we propose M3Cam, an innovative and lightweight SR imaging system for mobile phones. M3Cam can ensure high-quality 16x SR image (4x in both height and width) visualization with almost negligible latency. In detail, we utilize an optical image stabilization (OIS) module for lens control and introduce a new modality of data, namely gyroscope readings, to achieve high-precision and compact optical flow estimation modules. Building upon this concept, we design a multi-frame-based SR model utilizing the Swin Transformer. Our proposed system can generate a 16x SR image from four captured low-resolution images in real-time, with low computational load, low inference latency, and minimal reliance on runtime RAM. Through extensive experiments, we demonstrate that our proposed multi-modal optical flow model significantly enhances pixel alignment accuracy between multiple frames and delivers outstanding 16x SR imaging results under various shooting scenarios
Type
Publication
In Proceedings of the 22nd ACM Conference on Embedded Networked Sensor Systems